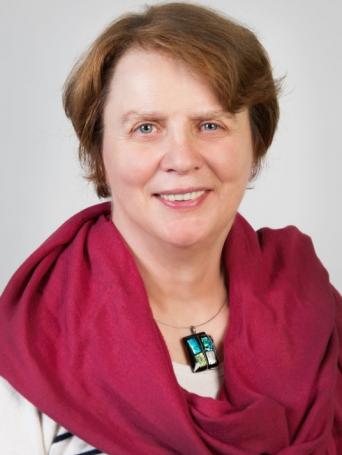
Julia Lane is a Professor at the NYU Wagner Graduate School of Public Service and a NYU Provostial Fellow for Innovation Analytics. She cofounded the Coleridge Initiative, whose goal is to use data to transform the way governments access and use data for the social good through training programs, research projects and a secure data facility
She currently serves on the Advisory Committee on Data for Evidence Building and the National AI Research Resources Task Force.
Previous to this, Julia was a Senior Managing Economist and Institute Fellow at American Institutes for Research. In this role Julia co-founded the Institute for Research on Innovation and Science (IRIS) at the University of Michigan. Julia has held positions at the National Science Foundation, The Urban Institute, The World Bank, American University and NORC at the University at Chicago.
In these positions, Julia has led many initiatives, including co-founding the Institute for Research and Innovation in Science (IRIS) at the University of Michigan and STAR METRICS programs at the National Science Foundation. She also initiated and led the creation and permanent establishment of the Longitudinal Employer-Household Dynamics Program at the U.S. Census Bureau. This program began as a small two year ASA Census Bureau fellowship and evolved into the first large-scale linked employer-employee dataset in the United States. It is now a permanent Census Bureau program with appropriated funds of $11 million per year.
Julia has published over 80 articles in leading economics journals, and authored or edited 13 books. She is an elected fellow of the American Association for the Advancement of Science, the International Statistical Institute and a fellow of the American Statistical Association. She has been the recipient of over $150 million in grants; from foundations such as the National Science Foundation, the Alfred P. Sloan Foundation, the Patrick J. McGovern Foundation, the Ewing Marion Kauffman Foundation, the MacArthur Foundation, the Russell Sage Foundation, the Spencer Foundation, the National Institutes of Health, the Overdeck Family Foundation, the Schmidt Futures Foundation and the Bill and Melinda Gates Foundation; from government agencies such as the Departments of Commerce, Labor, and Health and Human Services in the U.S., the ESRC in the U.K., and the Department of Labour and Statistics New Zealand in New Zealand, as well as from international organizations such as the World Bank. Julia is the recipient of the 2014 Julius Shiskin award and the 2014 Roger Herriot award. She is also the recipient of the 2017 Warren E. Miller Award. She was made a Distinguished Fellow of the New Zealand Association of Economists in 2019.
Julia received her PhD in Economics and Master's in Statistics from the University of Missouri.
The goal of this course is to develop the key data analytics skill sets necessary to inform evidence-based policy. Its design offers hands-on training in how to make sense of and use large scale real world heterogeneous datasets in the context of addressing real world problems. Students will learn how to scope a policy problem, understand the data generation process, how to manage, combine, and structure data, and how to create, measure and analyze the effect of different data decisions. They will also learn the basics of machine learning and visualization as well as inference, bias, privacy, and ethics issues. It is designed for graduate students who are seeking a stronger foundation in data analytics, although undergraduates with strong foundations in data and statistics will be considered for admission. The professor is a former senior White House advisor on the Federal Data Strategy and member of two White House Committees: the Advisory Committee on Data for Evidence Building and the National AI Research Resources Task Force.
The online textbook provides more information.
In a complex and difficult world, some idealism is needed to energize meaningful change. This course is for aspiring policy-makers who want to combine a necessary sense of optimism with real-world understanding of how to get things done. Each session will focus on specific examples of how practical solutions were found to seemingly intractable problems.
The goal of this course is to develop the key data analytics skill sets necessary to inform evidence-based policy. Its design offers hands-on training in how to make sense of and use large scale real world heterogeneous datasets in the context of addressing real world problems. Students will learn how to scope a policy problem, understand the data generation process, how to manage, combine, and structure data, and how to create, measure and analyze the effect of different data decisions. They will also learn the basics of machine learning and visualization as well as inference, bias, privacy, and ethics issues. It is designed for graduate students who are seeking a stronger foundation in data analytics, although undergraduates with strong foundations in data and statistics will be considered for admission. The professor is a former senior White House advisor on the Federal Data Strategy and member of two White House Committees: the Advisory Committee on Data for Evidence Building and the National AI Research Resources Task Force.
The online textbook provides more information.
The goal of this course is to develop the key data analytics skill sets necessary to inform evidence-based policy. Its design offers hands-on training in how to make sense of and use large scale real world heterogeneous datasets in the context of addressing real world problems. Students will learn how to scope a policy problem, understand the data generation process, how to manage, combine, and structure data, and how to create, measure and analyze the effect of different data decisions. They will also learn the basics of machine learning and visualization as well as inference, bias, privacy, and ethics issues. It is designed for graduate students who are seeking a stronger foundation in data analytics, although undergraduates with strong foundations in data and statistics will be considered for admission. The professor is a former senior White House advisor on the Federal Data Strategy and member of two White House Committees: the Advisory Committee on Data for Evidence Building and the National AI Research Resources Task Force.
The online textbook provides more information.
In a complex and difficult world, some idealism is needed to energize meaningful change. This course is for aspiring policy-makers who want to combine a necessary sense of optimism with real-world understanding of how to get things done. Each session will focus on specific examples of how practical solutions were found to seemingly intractable problems.
2024
2023
Profound changes in technology and climate, combined with the COVID-19 pandemic shock, have fundamentally changed the nature of work for firms and employees. And, because most labor markets are local, that change requires rethinking how data and evidence can be generated in a timely and actionable way to inform local decisions.
The emergence of new types of local data, new cloud-based platforms allowing state and local agencies to securely share de-identified confidential data, and new training programs to build state workforce capacity means that there is new potential for programs that are designed and shaped at the local level. A new workforce information system—a National Collaborative for Local Workforce Information (NCLWI)—can be designed that is driven by local needs and that is timely, actionable, and responsive.
This report describes why and how such a system should be constructed. The approach, inspired by the successful National Agricultural Extension Program, is fundamentally local in nature. It should be federally funded but driven by state and local needs, networks, and decision makers. It should build on the success of multistate data collaboratives in sharing data across agency and state lines and partnering with local universities. The result will be bottom-up, locally generated projects that can be tested, improved, and scaled to become products that can be put into practice across the country.
2022
The 2018 Nobel Laureate in Economic Sciences, Paul Romer of New York University speaks with New York University Professor Julia Lane. His work on endogenous technological change transformed our understanding of economic growth by recognizing that ideas—particularly scientific ideas—are neither a private nor a public good. Although he originally argued that the search for ideas is best fostered through competition, his thinking about the best structure has changed over time. In this interview he explains why.
This article describes how data science techniques—machine learning and natural language processing—can be used to open the black box of government data. It then describes how an incentive structure can be established—using human–computer interaction techniques —to create a new and sustainable data ecosystem. The particular focus is on the United States and on scientific researchers, who are major users of government data. However, the approach can be deployed to other use cases, such as data mentions in newspapers and government reports, and many other countries.
2020
2019
2018
Each issue of the Annals of the American Academy of Political and Social Science, guest edited by scholars and experts in the field, presents more than 200 pages of timely, in-depth research on a significant topic of interest to its readership which includes academics, researchers, policymakers, and professionals.
2017
The research community should move to fill the regulatory gap for large-scale social data left by the recent revisions of the Common Rule, argues Julia Ingrid Lane.
Big Data and Social Science: A Practical Guide to Methods and Tools shows how to apply data science to real-world problems in both research and the practice. The book provides practical guidance on combining methods and tools from computer science, statistics, and social science. This concrete approach is illustrated throughout using an important national problem, the quantitative study of innovation.
The text draws on the expertise of prominent leaders in statistics, the social sciences, data science, and computer science to teach students how to use modern social science research principles as well as the best analytical and computational tools. It uses a real-world challenge to introduce how these tools are used to identify and capture appropriate data, apply data science models and tools to that data, and recognize and respond to data errors and limitations.
The Jarmin and O'Hara piece describes a turning point in statistical data collection and dissemination. The federal agencies can no longer bear the full burden of producing and disseminating data for public policy research. Public policy researchers and schools should seize the new opportunity to complement the Census Bureau initiative—which also represents a turning point for both public policy education and research. We should adapt our educational programs so that we can produce the workforce capable of working with new data. We should adapt our research programs to move from largely artisanal individual efforts to large-scale “big science”; I have seen other scientific areas do so with great success.
We examine gender differences among the six PhD student cohorts 2004-2009 at the California Institute of Technology using a new dataset that includes information on trainees and their advisors and enables us to construct detailed measures of teams at the advisor level. We focus on the relationship between graduate student publications and: (1) their gender; (2) the gender of the advisor, (3) the gender pairing between the advisor and the student and (4) the gender composition of the team. We find that female graduate students co-author on average 8.5% fewer papers than men; that students writing with female advisors publish 7.7% more. Of particular note is that gender pairing matters: male students working with female advisors publish 10.0% more than male students working with male advisors; women students working with male advisors publish 8.5% less. There is no difference between the publishing patterns of male students working with male advisors and female students working with female advisors. The results persist and are magnified when we focus on the quality of the published articles, as measured by average Impact Factor, instead of number of articles. We find no evidence that the number of publications relates to the gender composition of the team. Although the gender effects are reasonably modest, past research on processes of positive feedback and cumulative advantage suggest that the difference will grow, not shrink, over the careers of these recent cohorts.
2015
In evaluating research investments, it is important to establish whether the expertise gained by researchers in conducting their projects propagates into the broader economy. For eight universities, it was possible to combine data from the UMETRICS project, which provided administrative records on graduate students supported by funded research, with data from the U.S. Census Bureau. The analysis covers 2010–2012 earnings and placement outcomes of people receiving doctorates in 2009–2011. Almost 40% of supported doctorate recipients, both federally and nonfederally funded, entered industry and, when they did, they disproportionately got jobs at large and high-wage establishments in high-tech and professional service industries. Although Ph.D. recipients spread nationally, there was also geographic clustering in employment near the universities that trained and employed the researchers. We also show large differences across fields in placement outcomes.
We examine gender differences among the six PhD student cohorts 2004–2009 at the California Institute of Technology using a new dataset that includes information on trainees and their advisors and enables us to construct detailed measures of teams at the advisor level. We focus on the relationship between graduate student publications and: (1) their gender; (2) the gender of the advisor, (3) the gender pairing between the advisor and the student and (4) the gender composition of the team. We find that female graduate students co-author on average 8.5% fewer papers than men; that students writing with female advisors publish 7.7% more. Of particular note is that gender pairing matters: male students working with female advisors publish 10.0% more than male students working with male advisors; women students working with male advisors publish 8.5% less. There is no difference between the publishing patterns of male students working with male advisors and female students working with female advisors. The results persist and are magnified when we focus on the quality of the published articles, as measured by average Impact Factor, instead of number of articles. We find no evidence that the number of publications relates to the gender composition of the team. Although the gender effects are reasonably modest, past research on processes of positive feedback and cumulative advantage suggest that the difference will grow, not shrink, over the careers of these recent cohorts.
Recent years have seen an increase in the amount of statistics describing different phenomena based on “Big Data.” This term includes data characterized not only by their large volume, but also by their variety and velocity, the organic way in which they are created, and the new types of processes needed to analyze them and make inference from them. The change in the nature of the new types of data, their availability, and the way in which they are collected and disseminated is fundamental. This change constitutes a paradigm shift for survey research. There is great potential in Big Data, but there are some fundamental challenges that have to be resolved before its full potential can be realized. This report provides examples of different types of Big Data and their potential for survey research; it also describes the Big Data process, discusses its main challenges, and considers solutions and research needs.
1990
1989
1988
1987
1986
1985
1983
2023 - 2024
KYStats MultiState PostSecondary Report2021 - 2022
Data as a Public Good2021 - 2023
ADRF Next Phase - Data Challenge, Expansion, and Sustainability2021 - 2022
Contract supplement2021 - 2022
Scaling Access to and Use of ERS data2021 - 2023
ADRF Next Phase - Data Challenge, Expansion, and Sustainability2021 - 2023
Contract supplement2021 - 2023
Scaling Access to and Use of ERS data2020 - 2020
Data platform for education and training data2020 - 2020
UI Claims in the COVID19 Era2020 - 2021
The Administrative Data Research Facility2019 - 2019
Federal Data Strategy2019 - 2019
Supplemental Award to 17610082019 - 2019
Workshop on Finding Datasets for Empirical Research in the Social Sciences2019 - 2019
Research funding, organizational context, and transformative research: New insights from new methods and data2019 - 2020
The Administrative Data Research Facility2019 - 2019
Transformative Data Initiative2019 - 2019
Data Science Training for Housing and Transportation Professionals in California: A UC-Berkeley Pilot2018 - 2018
Schmidt Sciences2018 - 2023
Increasing Accessibility and Value of USDA Food Data Resources2016 - 2018
Gates Foundation GrantGates Foundation Grant