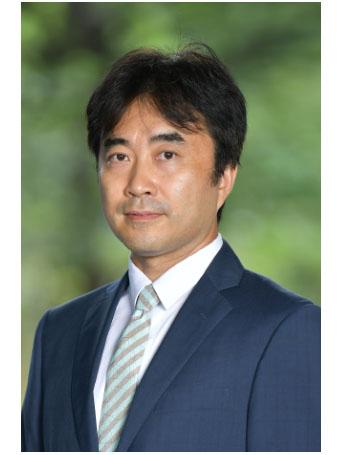
ChengHe Guan is Assistant Professor of Urban Science and Policy. He serves as a research consultant at the Centre on Migration, Policy and Society, University of Oxford and a senior visiting researcher at the School of Engineering and Applied Sciences, Harvard University. Dr. Guan is the co-director of NYU Shanghai Urban Lab. He received his master's and doctoral degrees from the Department of Urban Planning and Design, Harvard University. Dr. Guan’s research interests include (1) Computational urban science and space-time big data analytics; (2) Planning policy-informed urban growth simulation using CA and machine learning algorithm; and (3) Urban vital sign system and digital transformation of low-carbon smart cities. He has published more than 30 papers in internationally renowned journals indexed in SCI/SSCI. He has taught multiple courses “Urbanization in China”, “Planning Global Cities”, “Spatial Dynamics of Urbanization” and will teach a new course “Computational Urban Science”.
2021
COVID-19 was announced by the World Health Organization as a pandemic on March 11, 2020. Not only has COVID-19 struck the economy and public health, but it also has deep influences on people's feelings. Twitter, as an active social media, is a great database where we can investigate people's sentiments during this pandemic. By conducting sentiment analysis on Tweets using advanced machine learning techniques, this study aims to investigate how public sentiments respond to the pandemic from March 2 to May 21, 2020 in New York City, Los Angeles, London, and another six global mega-cities. Results showed that across cities, negative and positive Tweet sentiment clustered around mid-March and early May, respectively. Furthermore, positive sentiments of Tweets from New York City and London were positively correlated with stricter quarantine measures, although this correlation was not significant in Los Angeles. Meanwhile, Tweet sentiments of all three cities did not exhibit a strong correlation with new cases and hospitalization. Last but not least, we provide a qualitative analysis of the reasons behind differences in correlations shown above, along with a discussion of the polarizing effect of public policies on Tweet sentiments. Thus, the results of this study imply that Tweet sentiment is more sensitive to quarantine orders than reported statistics of COVID-19, especially in populous megacities where public transportation is heavily relied upon, which calls for prompt and effective quarantine measures during contagious disease outbreaks.
The rise of social networking platforms provides opportunities to examine the relationship between public emotion and housing price. This study investigates frequency and places of visits, population sentiment, and housing price using 8.7 million tweets retrieved from Manhattan, New York City in 2019. We implemented kernel density estimation, Getis-Ord Gi hot spot analysis, and spatial lagged hedonic pricing models to identify the location variation of sentiment levels. The results show: (1) the spatial clustering of tweets frequency was highly related to land use types in places such as parks, financial districts, and train stations; (2) high sentiment levels coincided with high frequency clusters and higher positive sentiment is associated with higher housing price; and (3) sentiment level was significantly associated with housing price and building structure, amenities, and proximity to landmarks all had significant influences on housing price. The study indicates that a population with higher concentration of happiness correlates to higher property value and provides an innovative perspective to understand public sentiment in relation to housing price using social media data, supplemented by housing transaction data. We demonstrate a feasible framework for researchers and stakeholders to utilize in future urban and spatial geographical research.
Urban green and open space are important components of achieving the goal of planning sustainable cities, by offering health benefits to urban dwellers and providing socio-economic and environmental benefits to society. Recent literature studied the usage of urban parks, however, few has addressed seasonal fluctuations of park visitor volume, let alone seasonal variations of home-park travel distances and park service areas. This paper not only empirically shows the seasonal variations of park visits but also examines links between the park visit patterns and spatial characteristics of the case parks. Applying spatial analysis methods to location data of over 1 million anonymous mobile phone samples collected from January to December 2011, we analyzed the seasonal variations in six medium-sized urban parks, of which size falls under the category of ‘district parks,’ in central Tokyo. We also conducted content analysis of a Japanese place review website to understand visitor perceptions of the case parks. On the other hand, park spatial characteristics data were collected and summarized through various ways including field observation and satellite image analysis. The results show that (1) while notable seasonal variations of park visitor volume and park service area existed in all case parks, the degree of variation also differed from park to park; (2) spatial characteristics of parks were closely interlinked to seasonal cultural events, to visitor perceptions, and consequently to seasonal fluctuations of the park visit patterns. Lessons learned from the policy perspective include highly diverse user groups visit these medium-sized urban parks than what the typical guidelines assume, and seasonal patterns of their visits considerably vary from park to park, interacting with spatial characteristics of the parks. Hence, the urban park planning process should consider specific and detailed characteristics of parks and allocate resources to respond to dynamic park visit patterns beyond generic guidelines.
A striking feature of urban formation has been the deployment of mega-blocks, often on the order of sixteen hectares or more. On the other hand, recent urban policies give strong suggestions for smaller and finer-grained neighborhood block and grid arrangements. This paper explores the transformation of urban block structures in high-density cities beyond spatial conditions of big versus small blocks by emphasizing “place” making through the degree of spatial diversity and flexibility. Using spatial indices of urban block arrangements, road network efficiencies and gradients of transit network accessibility, the assessment on urban neighborhood block structure is applied to territories of central core, suburban and peripheral development in Beijing, Shanghai and Shenzhen at multiple spatial scales. The results show that the overall efficiency and flexibility of urban block structures becomes more a matter of a narrowing of the range of differing block sizes among the three territories and a concomitant higher potential capacity for adaptation to a broader range of development options. Beyond the Chinese context, in high-density cities across the globe, policies on place making should adopt a multi-scale spatial analysis strategy to measure the configuration of the overall urban block structure and guide the transformation of the city.
With the availability of computational resources, geographical information systems, and remote sensing data, urban growth modeling has become a viable tool for predicting urbanization of cities and towns, regions, and nations around the world. This information allows policy makers, urban planners, environmental and civil organizations to make investments, design infrastructure, extend public utility networks, plan housing solutions, and mitigate adverse environmental impacts. Despite its importance, urban growth models often discard the spatiotemporal uncertainties in their prediction estimates. In this paper, we analyzed the uncertainty in the urban land predictions by comparing the outcomes of two different growth models, one based on a widely applied cellular automata model known as the SLEUTH CA and the other one based on a previously published machine learning framework. We selected these two models because they are complementary, the first is based on human knowledge and pre-defined and understandable policies while the second is more data-driven and might be less influenced by any a priori knowledge or bias. To test our methodology, we chose the cities of Jiaxing and Lishui in China because they are representative of new town planning policies and have different characteristics in terms of land extension, geographical conditions, growth rates, and economic drivers. We focused on the spatiotemporal uncertainty, understood as the inherent doubt in the predictions of where and when will a piece of land become urban, using the concepts of certainty area in space and certainty area in time. The proposed analyses in this paper aim to contribute to better urban planning exercises, and they can be extended to other cities worldwide.